Resolution
Veranova has taken steps to improve our ability to help customers achieve the ‘right first time’ and improve drug availability and time to market. We developed a global database of all our equipment including the vessels, impellers, baffles, cooling fluids, jacket design, etc. across the business. We utilized the Bourne protocol to understand the mixing time and length scale for the process chemistry and the API. A workflow was then developed to utilize mathematical modeling tools such as Dynochem®, Visimix®, & CFD (OpenFoam®).
Global database
Veranova’s global database includes detailed vessel dimensions and vessel drawings from across our sites, deployed in a basic web app using the Django framework. This app improves access to the equipment capacity, providing dimensions of the baffles, impellers and tanks and heat transfer for site engineers, technicians, and chemical development chemists. Using a unified database allows easier access to equipment geometry and configuration data so that the same data does not need to be gathered again and revalidated for each project to run in the specific reactor.
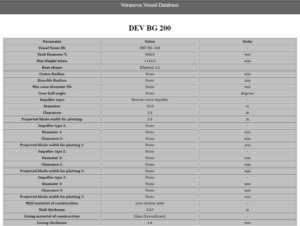
Figure 1: Veranova Global database in Django hosted on an Azure website
Traditional mixing scale-up
Traditional mixing scale-up is often based on a single parameter such as tip speed, power or volume . However, this approach is highly dependent on the application, mixing time and characteristic lengths. Critical process parameters for mixing are entirely different for hydrogenation, crystallization, or emulsification. Thus, there is not a ‘one-size-fits-all’ solution as equipment and process specifics must be considered. Also, it is very important to understand which mixing length and time scale (micro, meso, or macro) are limiting. While, historically, this traditional approach to scale-up may have worked in many instances, the traditional approach will fail on occasion as it is based on heuristics and generalizations, which may or may not apply to a specific process to be scaled. Therefore, the workflow presented below is employed to overcome the limitations associated with traditional scale-up methods.
Bourne protocol
Mixing is usually characterized by mixing length and time scales. It is extremely important to understand the most important mixing scale before tech transfer. Bourne2 protocol is utilized to narrow down the mixing timescale and length for the particular product and process. Macromixing would be at the scale of the tank with examples of uniform liquid blend time and solid distribution. Meso-mixing is at the scale of feed zone and anti-solvent plume in anti-solvent crystallization. Micro-mixing is at small scales of turbulence and diffusion time. Typical examples included solvent entrapment inside particles during fast precipitation.
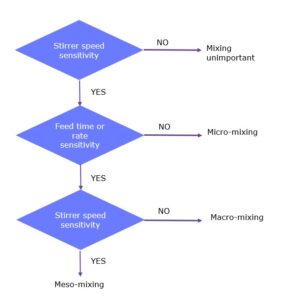
Figure 2: Bourne protocol for mixing and selectivity of chemical reactions
Workflow
The methodology we have developed utilizes the critical process metrics and scale-down experiments to de-risk scale-up. Three different software packages are utilized to improve our process understanding of mixing behavior across scale: Dynochem®, Vismix®, & CFD (OpenFOAM®). These packages differ in terms of complexity, accuracy, and simulation Cost. Dynochem® is the common tool within the business for mixing and scale-up. Critical Process Metrics (CPMs) in Dynochem are based on empirical correlations from the literature. Dynochem web utility for mixing has made it even easier to compare various reactors for tech transfer in terms of heat and mass transfer. Visimix® utilizes experimental data to predict the mixing performance across scales. Gas hold-up, specific mass transfer area, and specific mass transfer coefficient is predicted by Visimix® across the scale for hydrogenation reaction for reactor design selection. Maximum shear rates, max location concentration, and crystal collision energy obtained through Visimix are utilized for scale-up for crystallization. CFD is utilized to understand detailed flow patterns, vortex, shear rate, and distribution of multiphase volume fraction. Newer Lattice Boltzmann Method (LBM) CFD has been utilized to predict the droplet size distribution with good accuracy3–5. However, the final calculated values of mixing critical parameters such as gas phase mass transfer coefficient are based on the literature correlations.
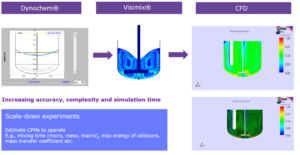
Figure 3: Workflow for mixing scale-up
Demonstration
This methodology has been utilized successfully to scale-up multiple products ‘right first time’. An example case study for the successful application of this methodology to a multiphase reaction (hydrogenation) was demonstrated across the scale. The reaction was scaled-up successfully from a lab-scale Parr (300 ml) reactor to a 100 gallon scale and finally to a 500 gallon reactor. Scale-down experiments were used to demonstrate control methodology and assumptions before scale-up. The total value of this product including multiple batches to the business was $3.35 m with the right quality specifications.
References:
- Kresta, S. M. & Brodkey, R. S. Turbulence in Mixing Applications. in Handbook of Industrial Mixing 19–87 (John Wiley & Sons, Ltd, 2003). doi:10.1002/0471451452.ch2.
- Bourne, J. R. Mixing and the Selectivity of Chemical Reactions. Org. Process Res. Dev. 7, 471–508 (2003).
- Thomas, J. A., DeVincentis, B., Wutz, J. & Ricci, F. Predicting the diameters of droplets produced in turbulent liquid–liquid dispersion. AIChE J. 68, e17667 (2022).
- Thomas, J. A., DeVincentis, B., Hanspal, N. & Kehn, R. O. Predicting gas-liquid mass transfer rates in reactors using a bubble parcel model. Chem. Eng. Sci. 264, 118183 (2022).
- Hanspal, N., DeVincentis, B. & Thomas, J. A. Modeling multiphase fluid flow, mass transfer, and chemical reactions in bioreactors using large-eddy simulation. Eng. Life Sci. 23, e2200020 (2023).